Self-tunable MPC and automatic coordinate discovery for flow control
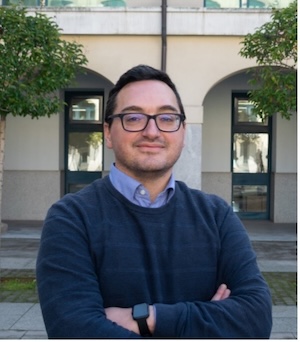
Time: Mon 2024-10-07 11.00 - 12.00
Location: Faxén, Teknikringen 8
Participating: Stefano Discetti (Univ. Carlos III, Madrid)
Abstract: This talk presents a noise-robust closed-loop control strategy for wake flows using model predictive control (MPC) and a data-driven approach for discovering low-dimensional actuation manifolds. We implemented a learning-based MPC framework which autonomously tunes hyperparameters through Bayesian optimization to maximize performance and robustness. The control achieves drag reduction and lift stabilization of the wake of a fluidic pinball, relying on aerodynamic force measurements. This approach demonstrates effective control with minimal user intervention. A methodology to identify meaningful low-dimensional embeddings with isometric mapping and to estimate flows with K-nearest neighbour regression is also presented. Although not yet integrated into the MPC, this manifold discovery reveals physically meaningful flow features that could inform future control strategies.
Bio: Stefano Discetti is Full Professor in the Department of Aerospace Engineering of Universidad Carlos III de Madrid. He received his Ph.D. in Aerospace Engineering from the University of Naples and was visiting researcher at Arizona State University. His research interests include non-intrusive measurement techniques in aerodynamics, machine learning-based flow control, and low-order modelling techniques. He received the Starting Grant of the European Research Council for his project NEXTFLOW, aiming to enhance optical flow diagnostics for control. He is a member of the Editorial Board of the JCR journal Measurement Science and Technology, the Steering Committee of the ERCOFTAC Special Interest Group in PIV, the Scientific Committee of the International PIV Symposia series, and the Scientific Council of the International Centre for Heat and Mass Transfer.